Igor Melnyk
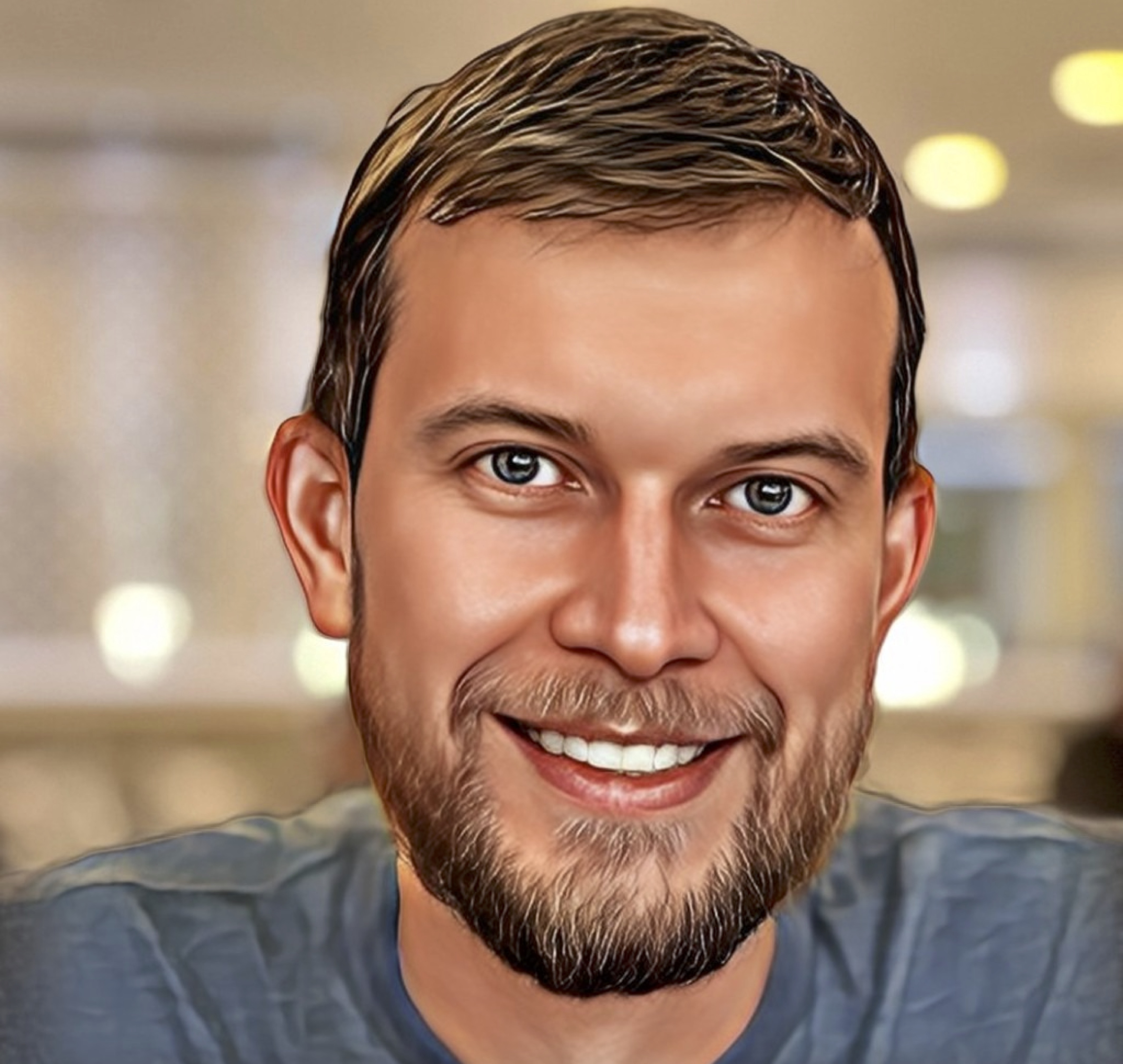
Office 32-134
1101 Kitchawan Rd
Yorktown Heights, NY
I am a Research Staff Member at IBM Thomas J. Watson Research Center, Yorktown Heights, NY working in the areas of Machine Learning and AI with the focus on Generative model in Natural Language Processing, Computer Vision and Biology.
I received a PhD in Computer Science and Engineering from University of Minnesota and MS in Computer Science from University of Colorado.
Earlier in my academic career, I have specialized in the field of robotics, working on collaborative localization of multi-robot systems. Building on this foundation, I then delved into the study of high-dimensional, heterogeneous time-series data streams and developed models and methods using Dynamic Bayesian Networks (DBN). Through my work, I proposed frameworks based on autoregressive and hidden Markov models to detect anomalies in multivariate time series data, and created efficient algorithms for learning and inference.
Upon joining IBM Research, I continued my journey in the domain of anomaly detection, applying my knowledge to several client projects with a focus on anomalous event detection and prediction. Over time, my research interests shifted towards deep learning generative models in Natural Language Processing (NLP) and Computer Vision. Drawing on my experience with dynamical Bayesian systems, I explored various modality transfers such as image-to-text systems (i.e., image captioning), text-to-knowledge graph transfers, and semantic style transfers between textual data.
Recently, I have become interested in the application of generative models in the biomedical domain, with a focus on protein and antibody design. The main guiding principle here is leveraging well-established pre-trained foundational models and re-prurposing them to the task at hand. For instance, I have proposed methods to distill AlphaFold’s metrics to develop more accurate and efficient inverse folding models. Additionally, I have explored the use of pre-trained language models for antibody sequence design using model reprogramming. I am also investigating the use of denoising-diffusion image models for conditional protein structure generation.